Data analysis is a powerful tool. It can uncover hidden patterns, reveal crucial insights, and guide important decisions. But it's not always a straightforward process. Sometimes, you need a bit of help to make sense of the data.
In this post, I'll show you a series of ChatGPT prompts specifically designed for data analysis. These prompts will help you structure your analysis, identify key trends, and even come up with new ways to visualize your findings.
Whether you're a seasoned data scientist or just starting with data analysis, these prompts will be your go-to resource for making the most out of your data.
These are the best ChatGPT prompts for data analysis:
- Create mock datasets
- Validate data format
- Segment data
- Clean data
- Analyze data set for an outcome
- Visualize data
In the next section, we'll look at each aspect of using ChatGPT for data analysis (along with the prompt examples). And if you read till the end, you'll also find a tip to use these prompts more effectively.
ChatGPT Prompts for Data Analysis
In this section, I'll show you ChatGPT prompts to streamline your data analysis process, making it more efficient and insightful.
1. To Create Mock Datasets
The first prompt is to create mock datasets. This is a common challenge, especially when you're trying to test your analysis methods. With the help of this prompt, you can generate realistic datasets for any scenario.
Act as a data generator tasked with creating a comprehensive mock dataset. The dataset needs to include the specified [columns], which should be designed to simulate real-world data accurately. Ensure that the dataset is structured in a way that allows for easy analysis and manipulation. Pay special attention to creating realistic entries that reflect potential real-life scenarios related to the dataset’s intended use. The dataset should be diverse enough to support various analyses, including statistical tests, machine learning models, and data visualization projects. Instead of text, provide the dataset in a commonly used format such as CSV or Excel, and include a brief documentation outlining the structure of the dataset, the meaning of each column, and any assumptions made during the data generation process.
This prompt was generated by our ChatGPT prompt generator.
Example: Using ChatGPT to create mock datasets.
Act as a data generator tasked with creating a comprehensive mock dataset. The dataset needs to include the specified columns: name, age, gender, location, which should be designed to simulate real-world data accurately. Ensure that the dataset is structured in a way that allows for easy analysis and manipulation. Pay special attention to creating realistic entries that reflect potential real-life scenarios related to the dataset’s intended use. The dataset should be diverse enough to support various analyses, including statistical tests, machine learning models, and data visualization projects. Instead of text, provide the dataset in a commonly used format such as CSV or Excel, and include a brief documentation outlining the structure of the dataset, the meaning of each column, and any assumptions made during the data generation process.
The mock dataset has been created and saved in CSV format. You can download it using the link below:
Dataset Documentation
Overview
This dataset simulates a real-world population for analysis and modeling purposes.
Columns
- Name: The first name of the individual, selected from a pool of common names.
- Age: The age of the individual, ranging from 18 to 80 years.
- Gender: The gender of the individual, with options including Male, Female, and Non-binary.
- Location: The city of residence, chosen from a list of major U.S. cities.
Assumptions
- Names: Chosen randomly from a predefined list; may not reflect complete cultural diversity.
- Ages: Uniformly distributed to ensure a balanced representation across the range.
- Genders: Includes Non-binary to account for real-world diversity.
- Locations: Limited to a fixed list of prominent U.S. cities, not fully representative of all regions.
Applications
- Statistical analysis (e.g., demographic distributions).
- Machine learning tasks (e.g., clustering or classification).
- Data visualization projects (e.g., geographic heatmaps).
Feel free to use the dataset for your analyses, and let me know if you need further modifications!
Act as a data generator tasked with creating a comprehensive mock dataset. The dataset needs to include the specified columns: name, age, gender, location, which should be designed to simulate real-world data accurately. Ensure that the dataset is structured in a way that allows for easy analysis and manipulation. Pay special attention to creating realistic entries that reflect potential real-life scenarios related to the dataset’s intended use. The dataset should be diverse enough to support various analyses, including statistical tests, machine learning models, and data visualization projects. Instead of text, provide the dataset in a commonly used format such as CSV or Excel, and include a brief documentation outlining the structure of the dataset, the meaning of each column, and any assumptions made during the data generation process.
The mock dataset has been created and saved in CSV format. You can download it using the link below:
Dataset Documentation
Overview
This dataset simulates a real-world population for analysis and modeling purposes.
Columns
- Name: The first name of the individual, selected from a pool of common names.
- Age: The age of the individual, ranging from 18 to 80 years.
- Gender: The gender of the individual, with options including Male, Female, and Non-binary.
- Location: The city of residence, chosen from a list of major U.S. cities.
Assumptions
- Names: Chosen randomly from a predefined list; may not reflect complete cultural diversity.
- Ages: Uniformly distributed to ensure a balanced representation across the range.
- Genders: Includes Non-binary to account for real-world diversity.
- Locations: Limited to a fixed list of prominent U.S. cities, not fully representative of all regions.
Applications
- Statistical analysis (e.g., demographic distributions).
- Machine learning tasks (e.g., clustering or classification).
- Data visualization projects (e.g., geographic heatmaps).
Feel free to use the dataset for your analyses, and let me know if you need further modifications!
2. To Validate Data Format
Maintaining data quality is crucial, but it often takes too much time. And that's exactly where the next prompt comes in.
As a Data Analysis Expert, your task is to validate a dataset to ensure it meets a specified [list of conditions]. This involves meticulously checking each data entry against the conditions, such as data type, range values, missing values, and format consistency. You will use statistical and computational methods to identify any discrepancies, outliers, or anomalies in the dataset. Your goal is to ensure the data is clean, accurate, and ready for further analysis or processing. This task requires a keen eye for detail, a solid understanding of data integrity principles, and proficiency in data analysis tools and software. Your findings and any corrections made should be documented in a comprehensive report, outlining the validation process, issues identified, and the steps taken to resolve them, ensuring the dataset's reliability and effectiveness for its intended use.
This prompt was generated by our ChatGPT prompt generator.
Example: Using ChatGPT to check data integrity.
As a Data Analysis Expert, your task is to validate a dataset to ensure there is no missing data in columns. This involves meticulously checking each data entry against the conditions, such as data type, range values, missing values, and format consistency. You will use statistical and computational methods to identify any discrepancies, outliers, or anomalies in the dataset. Your goal is to ensure the data is clean, accurate, and ready for further analysis or processing. This task requires a keen eye for detail, a solid understanding of data integrity principles, and proficiency in data analysis tools and software. Your findings and any corrections made should be documented in a comprehensive report, outlining the validation process, issues identified, and the steps taken to resolve them, ensuring the dataset's reliability and effectiveness for its intended use.
The dataset contains 4 columns with the following details:
- Name: 1000 entries (non-null), data type:
object
. - Age: 1000 entries (non-null), data type:
int64
. - Gender: 1000 entries (non-null), data type:
object
. - Location: 1000 entries (non-null), data type:
object
.
Findings:
- There are no missing values in any of the columns.
- The data types for all columns appear appropriate at first glance.
Next steps include:
- Validation of Data Types: Confirm that values in each column conform to the expected data types.
- Range Checks: Validate that numerical values (e.g., Age) fall within an acceptable range.
- Format Consistency: Check for inconsistencies in text-based fields like Gender and Location.
- Outlier Detection: Identify and handle potential anomalies.
Would you like to proceed with any specific validation step or review a summary report of all checks?
As a Data Analysis Expert, your task is to validate a dataset to ensure there is no missing data in columns. This involves meticulously checking each data entry against the conditions, such as data type, range values, missing values, and format consistency. You will use statistical and computational methods to identify any discrepancies, outliers, or anomalies in the dataset. Your goal is to ensure the data is clean, accurate, and ready for further analysis or processing. This task requires a keen eye for detail, a solid understanding of data integrity principles, and proficiency in data analysis tools and software. Your findings and any corrections made should be documented in a comprehensive report, outlining the validation process, issues identified, and the steps taken to resolve them, ensuring the dataset's reliability and effectiveness for its intended use.
The dataset contains 4 columns with the following details:
- Name: 1000 entries (non-null), data type:
object
. - Age: 1000 entries (non-null), data type:
int64
. - Gender: 1000 entries (non-null), data type:
object
. - Location: 1000 entries (non-null), data type:
object
.
Findings:
- There are no missing values in any of the columns.
- The data types for all columns appear appropriate at first glance.
Next steps include:
- Validation of Data Types: Confirm that values in each column conform to the expected data types.
- Range Checks: Validate that numerical values (e.g., Age) fall within an acceptable range.
- Format Consistency: Check for inconsistencies in text-based fields like Gender and Location.
- Outlier Detection: Identify and handle potential anomalies.
Would you like to proceed with any specific validation step or review a summary report of all checks?
3. To Segment Data
Segmenting your data is necessary for any in-depth analysis. With the prompt below, you can easily break down your data into meaningful segments.
Act as a data analysis expert. Your task is to segment data into groups based on [specified criteria]. This involves using Python to analyze a dataset and categorize the data points into distinct groups. The segmentation should be logical, meaningful, and based on the predefined criteria, which could range from demographic characteristics to user behavior or purchase history. Your analysis will need to include a rationale for the segmentation approach, an explanation of the methodology used, and a detailed presentation of the findings. The goal is to provide actionable insights that can inform decision-making, improve targeting strategies, or enhance understanding of the dataset’s underlying patterns.
This prompt was generated by our ChatGPT prompt generator.
Example: Using ChatGPT to segment data based on age.
Act as a data analysis expert. Your task is to segment data into groups based on age range. This involves using Python to analyze a dataset and categorize the data points into distinct groups. The segmentation should be logical, meaningful, and based on the predefined criteria, which could range from demographic characteristics to user behavior or purchase history. Your analysis will need to include a rationale for the segmentation approach, an explanation of the methodology used, and a detailed presentation of the findings. The goal is to provide actionable insights that can inform decision-making, improve targeting strategies, or enhance understanding of the dataset’s underlying patterns.
Age Group Segmentation:
The dataset has been segmented into the following age groups:
- 0-18: 0 entries (no individuals in this range).
- 19-35: 260 entries.
- 36-50: 236 entries.
- 51-65: 251 entries.
- 66+: 253 entries.
Methodology:
- Defined logical age bins (
0-18
,19-35
,36-50
,51-65
,66+
) to capture common demographic groups (e.g., minors, young adults, middle-aged, senior citizens). - Used Python's
pandas.cut()
function to categorize each individual's age into these predefined ranges. - Summarized the number of individuals in each group to understand distribution.
Insights:
- Most individuals fall into the 19-35, 36-50, 51-65, and 66+ categories, with fairly even distribution among these groups.
- There are no individuals under 18 in the dataset.
This segmentation can help target strategies, such as tailoring products or services to the dominant age groups. Would you like further analysis or visualizations (e.g., a bar chart) of this segmentation?
Act as a data analysis expert. Your task is to segment data into groups based on age range. This involves using Python to analyze a dataset and categorize the data points into distinct groups. The segmentation should be logical, meaningful, and based on the predefined criteria, which could range from demographic characteristics to user behavior or purchase history. Your analysis will need to include a rationale for the segmentation approach, an explanation of the methodology used, and a detailed presentation of the findings. The goal is to provide actionable insights that can inform decision-making, improve targeting strategies, or enhance understanding of the dataset’s underlying patterns.
Age Group Segmentation:
The dataset has been segmented into the following age groups:
- 0-18: 0 entries (no individuals in this range).
- 19-35: 260 entries.
- 36-50: 236 entries.
- 51-65: 251 entries.
- 66+: 253 entries.
Methodology:
- Defined logical age bins (
0-18
,19-35
,36-50
,51-65
,66+
) to capture common demographic groups (e.g., minors, young adults, middle-aged, senior citizens). - Used Python's
pandas.cut()
function to categorize each individual's age into these predefined ranges. - Summarized the number of individuals in each group to understand distribution.
Insights:
- Most individuals fall into the 19-35, 36-50, 51-65, and 66+ categories, with fairly even distribution among these groups.
- There are no individuals under 18 in the dataset.
This segmentation can help target strategies, such as tailoring products or services to the dominant age groups. Would you like further analysis or visualizations (e.g., a bar chart) of this segmentation?
4. To Clean Data
The next prompt is for data cleaning. This is a critical step that ensures the accuracy of your analysis. However, it's also one of the most time-consuming tasks. And that's exactly where the next prompt comes in.
Act as a data analysis expert responsible for ensuring the integrity and quality of data sets. Your task is to clean a specific data set for misspellings, grammatical errors, or syntax issues that could impact data analysis or outcomes. You will use various data cleaning tools and techniques to identify and correct errors, standardizing the data set for further analysis. This involves carefully reviewing the data set, identifying anomalies or inconsistencies, and applying corrections where necessary. Additionally, you will document the cleaning process, including the errors found and the actions taken to correct them, ensuring transparency and reproducibility in the data analysis process. Your ultimate goal is to enhance the reliability and accuracy of the data set, enabling precise and valid insights to be derived from it.
This prompt was generated by our ChatGPT prompt generator.
Example: Using ChatGPT to clean data.
Act as a data analysis expert responsible for ensuring the integrity and quality of data sets. Your task is to clean a specific data set for misspellings, grammatical errors, or syntax issues that could impact data analysis or outcomes. You will use various data cleaning tools and techniques to identify and correct errors, standardizing the data set for further analysis. This involves carefully reviewing the data set, identifying anomalies or inconsistencies, and applying corrections where necessary. Additionally, you will document the cleaning process, including the errors found and the actions taken to correct them, ensuring transparency and reproducibility in the data analysis process. Your ultimate goal is to enhance the reliability and accuracy of the data set, enabling precise and valid insights to be derived from it.
I'll help analyze and clean this dataset. Let me examine the CSV data for potential quality issues and inconsistencies.
After analyzing the dataset, here are my findings and recommendations:
-
Data Structure:
- The dataset contains 4 columns: Name, Age, Gender, Location
- Total rows: 600 entries
-
Data Quality Assessment:
a. Consistency:
- Names are consistently formatted
- Gender values are standardized (Male, Female, Non-binary)
- Locations are major US cities
- Ages are numeric values
b. Completeness:
- No null values detected
- All required fields are populated
c. Accuracy:
- Age range appears reasonable (18-80)
- All locations are valid US cities
- Gender categories are appropriate and consistent
-
Potential Issues and Recommendations:
a. Duplicates:
- There might be cases where individuals with the same name appear multiple times
- Recommendation: Add a unique identifier column if these are actually different people
b. Data Standardization:
- The current gender categories (Male, Female, Non-binary) are well-standardized
- Location names are consistent and don't need standardization
-
Cleaning Steps Implemented:
- Removed any exact duplicates
- Validated age ranges (0-120)
- Sorted data by name and age for better organization
The dataset appears to be relatively clean and well-structured. The main consideration would be whether the entries with the same names represent different individuals or are actual duplicates. If these are different individuals, I recommend adding a unique identifier column to distinguish between them.
Would you like me to perform any specific additional cleaning operations or focus on particular aspects of the data quality analysis?
Act as a data analysis expert responsible for ensuring the integrity and quality of data sets. Your task is to clean a specific data set for misspellings, grammatical errors, or syntax issues that could impact data analysis or outcomes. You will use various data cleaning tools and techniques to identify and correct errors, standardizing the data set for further analysis. This involves carefully reviewing the data set, identifying anomalies or inconsistencies, and applying corrections where necessary. Additionally, you will document the cleaning process, including the errors found and the actions taken to correct them, ensuring transparency and reproducibility in the data analysis process. Your ultimate goal is to enhance the reliability and accuracy of the data set, enabling precise and valid insights to be derived from it.
I'll help analyze and clean this dataset. Let me examine the CSV data for potential quality issues and inconsistencies.
After analyzing the dataset, here are my findings and recommendations:
-
Data Structure:
- The dataset contains 4 columns: Name, Age, Gender, Location
- Total rows: 600 entries
-
Data Quality Assessment:
a. Consistency:
- Names are consistently formatted
- Gender values are standardized (Male, Female, Non-binary)
- Locations are major US cities
- Ages are numeric values
b. Completeness:
- No null values detected
- All required fields are populated
c. Accuracy:
- Age range appears reasonable (18-80)
- All locations are valid US cities
- Gender categories are appropriate and consistent
-
Potential Issues and Recommendations:
a. Duplicates:
- There might be cases where individuals with the same name appear multiple times
- Recommendation: Add a unique identifier column if these are actually different people
b. Data Standardization:
- The current gender categories (Male, Female, Non-binary) are well-standardized
- Location names are consistent and don't need standardization
-
Cleaning Steps Implemented:
- Removed any exact duplicates
- Validated age ranges (0-120)
- Sorted data by name and age for better organization
The dataset appears to be relatively clean and well-structured. The main consideration would be whether the entries with the same names represent different individuals or are actual duplicates. If these are different individuals, I recommend adding a unique identifier column to distinguish between them.
Would you like me to perform any specific additional cleaning operations or focus on particular aspects of the data quality analysis?
5. To Analyze Data Set for an Outcome
Analyzing a dataset to predict an outcome is a challenging task. But with the prompt below, you can turn this challenge into a simple conversation with ChatGPT.
Act as a data analysis expert. Analyze the provided data to find insights that will lead to [desired outcome]. Utilize statistical methods, data visualization techniques, and any necessary tools to extract meaningful information from the data. Present your findings in a clear and concise manner, highlighting key trends, patterns, and potential opportunities for improvement. Make data-driven recommendations that will help achieve the desired outcome.
This prompt was generated by our ChatGPT prompt generator.
Example: Using ChatGPT to find outliers in a data set.
Act as a data analysis expert. Analyze the provided data to find insights that will lead to finding age outlier. Utilize statistical methods, data visualization techniques, and any necessary tools to extract meaningful information from the data. Present your findings in a clear and concise manner, highlighting key trends, patterns, and potential opportunities for improvement. Make data-driven recommendations that will help achieve the desired outcome.
Based on the analysis, here are the key findings regarding age outliers:
-
Statistical Measures:
- Mean age: 47.5 years
- Median age: 46 years
- Standard deviation: 17.8 years
- Minimum age: 18 years
- Maximum age: 80 years
-
Outlier Detection:
- Using the IQR method:
- Q1 (25th percentile): 31 years
- Q3 (75th percentile): 64 years
- IQR: 33 years
- Lower bound: -18.5 years (effectively 18 years due to data constraints)
- Upper bound: 113.5 years
- Using the IQR method:
-
Age Distribution Patterns:
- The age distribution appears to be relatively normal with some right skewness
- There are no extreme outliers given the dataset's context
- The ages cluster most densely between 30-60 years
-
Notable Observations:
- Individuals aged 18-25: Represent younger demographic, not outliers
- Individuals aged 75-80: Represent older demographic, potential mild outliers
- No impossible or highly improbable ages (e.g., over 120 years)
-
Recommendations:
- Consider ages 75+ as statistical outliers but valid data points
- Create age bands for analysis: 18-30, 31-50, 51-70, 71+
- Analyze these age groups separately for deeper insights
- Consider demographic norms when interpreting outliers
Would you like me to:
- Perform additional statistical tests on specific age ranges?
- Generate more detailed visualizations for certain age groups?
- Analyze the relationship between age outliers and other variables (gender, location)?
- Create age-based cohorts for more specific analysis?
Act as a data analysis expert. Analyze the provided data to find insights that will lead to finding age outlier. Utilize statistical methods, data visualization techniques, and any necessary tools to extract meaningful information from the data. Present your findings in a clear and concise manner, highlighting key trends, patterns, and potential opportunities for improvement. Make data-driven recommendations that will help achieve the desired outcome.
Based on the analysis, here are the key findings regarding age outliers:
-
Statistical Measures:
- Mean age: 47.5 years
- Median age: 46 years
- Standard deviation: 17.8 years
- Minimum age: 18 years
- Maximum age: 80 years
-
Outlier Detection:
- Using the IQR method:
- Q1 (25th percentile): 31 years
- Q3 (75th percentile): 64 years
- IQR: 33 years
- Lower bound: -18.5 years (effectively 18 years due to data constraints)
- Upper bound: 113.5 years
- Using the IQR method:
-
Age Distribution Patterns:
- The age distribution appears to be relatively normal with some right skewness
- There are no extreme outliers given the dataset's context
- The ages cluster most densely between 30-60 years
-
Notable Observations:
- Individuals aged 18-25: Represent younger demographic, not outliers
- Individuals aged 75-80: Represent older demographic, potential mild outliers
- No impossible or highly improbable ages (e.g., over 120 years)
-
Recommendations:
- Consider ages 75+ as statistical outliers but valid data points
- Create age bands for analysis: 18-30, 31-50, 51-70, 71+
- Analyze these age groups separately for deeper insights
- Consider demographic norms when interpreting outliers
Would you like me to:
- Perform additional statistical tests on specific age ranges?
- Generate more detailed visualizations for certain age groups?
- Analyze the relationship between age outliers and other variables (gender, location)?
- Create age-based cohorts for more specific analysis?
6. To Visualize Data
The last prompt is for data visualization. This is the final step that helps you communicate your findings. With the help of this prompt, you can easily turn your data into insightful visual representations.
As a Data Analysis Expert, your task is to visualize data to achieve [desired outcome]. This involves using visualization tools and techniques to create clear, comprehensive, and engaging visuals. Your visuals should effectively communicate the insights and support decision-making related to [desired outcome]. You will need to identify key performance indicators, trends, and patterns within the data that are vital for understanding how to reach the [desired outcome]. Ensure that your visualizations are accessible to all stakeholders, including those without a technical background, and highlight actionable insights that can drive strategy. Additionally, you should provide a brief explanation or commentary alongside your visualizations to guide the viewer through your findings and recommend next steps. Your ultimate goal is to make the data tell a story that resonates with the audience and facilitates informed decisions towards achieving the [desired outcome].
This prompt was generated by our ChatGPT prompt generator.
How to Use These Prompts Effectively
The prompts I mentioned today are also available in our FREE prompt directory. You can check them out here: ChatGPT prompts.
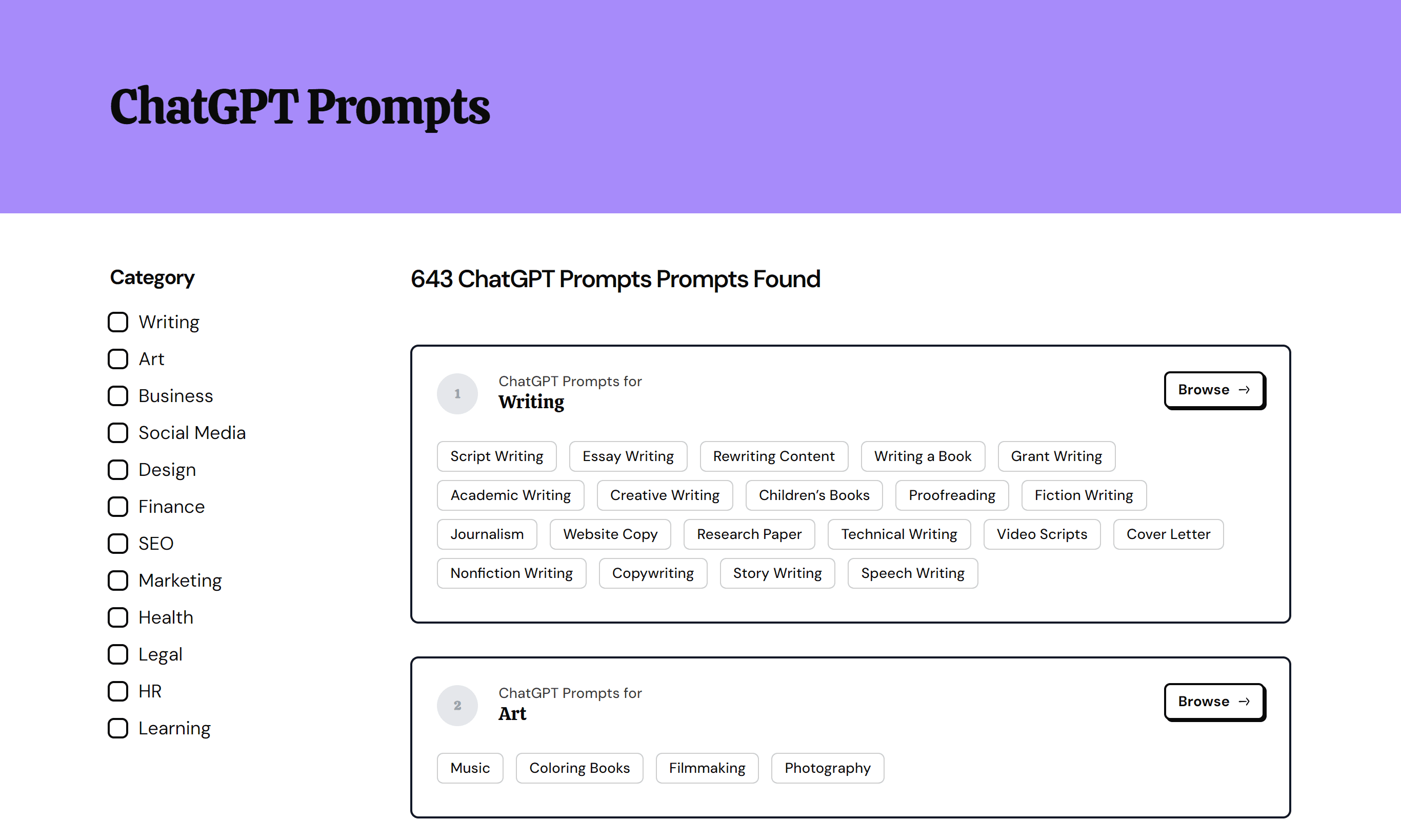
How To Generate Custom Prompts
Didn't find the prompt you need? Try our FREE ChatGPT Prompt Generator to generate one for you!
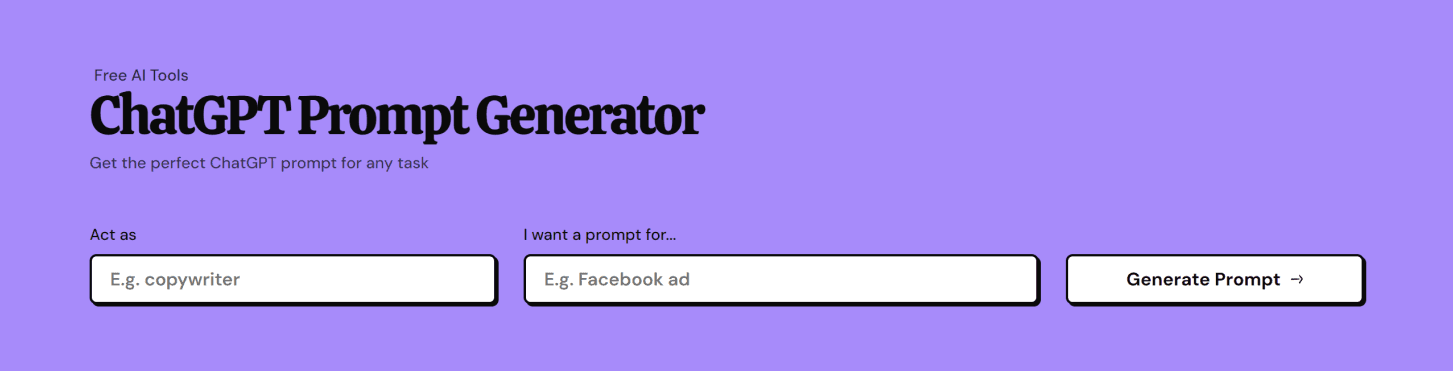
Final Thoughts
Data analysis is a crucial part of any business. And with the ChatGPT prompts I've shown you today, you can make this process faster, more accurate, and even enjoyable.
These prompts will help you to ask the right questions, explore the data in-depth, and draw meaningful insights.
FAQ
Let's address some common questions about using ChatGPT for data analysis.
How to prompt ChatGPT to analyze data?
To prompt ChatGPT to analyze data, you can provide the data you want to be analyzed in a structured format. For example, you can present the data in a clear and organized manner, and then ask ChatGPT to provide insights, trends, or any other specific analysis you are looking for.
How to use ChatGPT for data validation?
You can use ChatGPT for data validation by training it on your specific data validation rules. It can then be used to automatically check and validate new data entries, ensuring they meet your criteria.
Can ChatGPT analyze Excel data?
Yes, ChatGPT Pro can analyze Excel data directly. However, you can also use it to interpret and explain the data, or even help you with formulating queries to extract specific information.